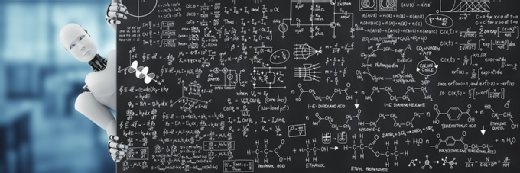
Blue Planet Studio - stock.adobe
AI firm saves a million in shift to Pure FlashBlade shared storage
AI consultancy Crater Labs spent vast amounts of time managing server-attached drives to ensure GPUs were saturated. A shift to all-flash Pure Storage slashed that to almost zero
Toronto-based artificial intelligence (AI) consultancy Crater Labs has saved around CAN$1.5m (£885,000) in researcher time after it replaced difficult-to-configure direct-attached storage with shared capacity in a FlashBlade array from Pure Storage.
The move saw it virtually eliminate the need for its researchers to spend time configuring storage infrastructure for AI training runs on customer projects.
Crater Labs supplies proof-of-concept and research work in AI for its customers. This focuses on the training phase of AI, upon completion of which projects are handed back to the customer.
Experiments it has worked on for customers include developing AI to: detect defects in manufacturing processes; analyse SEC data in three days instead of 10; calculate delivery routes for thousands of trucks in two-thirds less time; and detect billing anomalies with up to 93% accuracy for telco and utility companies.
“Customers may do AI/ML processing but are not able to do research in-house to develop something that’s not available off the shelf,” said Khalid Eidoo, founder and CEO at Crater Labs. “Customers bring us in to develop new models based on the latest that’s coming out of academia.”
Previously, Crater Labs worked in the cloud or on in-house directed-attached flash and spinning disk in servers.
Running AI in the cloud proved costly for the company, said Eidoo. “Our projects are often datasets of multiple terabytes, and training in the cloud was not the most practical thing,” he said. “Datasets are diverse because we have multiple projects running for customers simultaneously, which can mean many file types and sizes, and that brought restrictions in how we could interact with services from the cloud provider.”
Read more on AI and data storage
- Storage technology explained: AI and data storage. In this guide, we examine the data storage needs of artificial intelligence, the demands it places on data storage, the suitability of cloud and object storage for AI, and key AI storage products.
- Podcast: What is the impact of AI on storage and compliance? Start now looking at artificial intelligence compliance. That’s the advice of Mathieu Gorge of Vigitrust, who says AI governance is still immature but firms should recognise the limits and still act.
In-house, the limits came when trying to feed multiple models in parallel to heterogeneous storage media split across multiple servers.
“There could be 12 projects at a time, and our researchers needed to configure storage for them,” said Eidoo. “Data types can range from very large images to databases, all with very different I/O [input/output] signatures.
“Because each server had its own storage, there was a lot of shuffling of data to the right place, but still we often couldn’t saturate the GPUs [graphics processing units],” he said. “We didn’t want to have to deal with all that. It was taking our researchers three or four days to configure storage for each experiment.”
Crater Labs therefore switched to Pure Storage FlashBlade, which targets unstructured data in file and block storage workloads and comes with TLC or QLC (higher capacity) flash drives.
Crater Labs runs about 127TB of FlashBlade capacity that provides storage capacity via Ethernet to Linux-based AI server clusters that run “several dozen” Nvidia GPUs. AI workloads are spun up via containers, which are easily provisioned with storage capacity.
Key among the benefits are that researchers now do not have to spend time setting up storage for each AI training run. “It took researchers about 10% of the time spent on each project to work on infrastructure-related tasks,” said Eidoo. “That’s virtually eliminated now. We don’t think twice about data location.”
He said that meant the time to train models has dropped by between 25% and 40%. “That means the team isn’t sitting for two or three weeks waiting around,” said Eidoo. “Multiply that across 12 experiments and four to six researchers, and that’s a pretty big multiplier effect. We’re saving close to CAN$1.5m not having to spend time setting up infrastructure.”